By Jason Lancaster
Winston Churchill once stated that the only thing that scared him during the Second World War was the subsea aspect during the Battle of the Atlantic. Ant-submarine Warfare (ASW) was a paramount focus early in the war. Victory in the Atlantic required a whole of government effort from the Allied powers at the war’s strategic, operational, and tactical levels. At the strategic level, national shipbuilding industries designed ships like the Flower-class corvette and Liberty cargo ship for mass production. At the operational and tactical level, Allied air forces and navies were forced to operate jointly to hunt submarines and defend convoys.
During the Cold War, NATO maintained anti-submarine competency and internalized lessons learned during the Second World War. The collapse of the Soviet Navy during the 1990s shifted the U.S. focus to power projection ashore in the Balkans and the Middle East, and anti-submarine warfare competencies across the joint force atrophied. As the era of near-peer competition began, the Navy looked at ways to recapture the hard-fought competencies and lessons lost since the end of the Cold War. In particular, the whole government approach to anti-submarine warfare (ASW) was reintroduced, known as “full-spectrum ASW.” The Navy is the domain owner for undersea warfare. As such, the Navy must be prepared to educate and explain undersea warfare doctrine and its roles to the rest of the joint force so that lessons written in blood are not repeated.
A Disappointing Tale of Disjointedness
As a young lieutenant serving at Naval Forces in Korea, I received a frantic call from a United States Forces Korea staff officer to come over for a chat. Once there, an Air Force officer asked me how to make anti-submarine warfare a joint activity. Inadvertently, my recommendations aligned with the concepts of “full-spectrum ASW,” first described by retired Navy captain William Toti. During the meeting, I detailed several ways to accomplish anti-submarine warfare as a joint activity. A few hours later, I received a call from an Air Force officer stating that headquarters decided that anti-submarine warfare was a Navy problem, a position reminiscent of the historical friction between the Army Air Corps and the Navy in 1942. History has a way of repeating itself.
Anti-Submarine Warfare (ASW) is All-of-Government and Joint
Full-spectrum ASW breaks down the different ways to defeat a submarine threat. There are offensive and defensive lines of effort or threads. The threads cover the strategic, operational, and tactical levels of war. These threads require an all-of-government approach to recognize their full benefit and potential. Captain Toti was inspired by the Army’s combined arms tactics and use of kill zones to approach anti-submarine warfare. Using my previously mentioned conversation as an inspiration, this paper journeys through the different threads of integrating full-spectrum ASW into the joint force and not leaving it just to the Navy.
Strategic Threads
The joint force contributes variously at the levels of war for full-spectrum ASW. There are only two threads at the strategic level of war. If used aggressively by joint force commanders, those two threads can end a submarine threat before it has begun.
1. Discourage enemy submarines before leaving the harbor. Discouraging enemy submarines from leaving port occurs at senior levels of government and the combatant command through overt and covert means. Commanders and the government should conduct studies to determine enemy submarine force capability gaps to exploit. This should be all-encompassing and explore everything from adversary submarine force leadership, command and control, tactics, training, parts and maintenance, and morale. Discouraging an enemy submarine from leaving port would focus on deterring its leadership, disrupting command and control, and exploiting any issues with submarine force morale. Many of these efforts can be considered part of information operations or operations designed to influence the decision calculus of enemy leadership and crews to create favorable outcomes for the joint forces.
2. Defeat submarines in port. The adage is that the best time to eliminate a submarine is when it’s stationary alongside a pier. In 1982, the ARS Santa Fe was sunk alongside a pier on South Georgia Island by a Royal Navy helicopter. This reduced the Argentine Navy’s submarine fleet by a third before the amphibious landings in the Falklands began. The remaining two Argentine submarines proved troublesome for the Royal Fleet. The Royal Navy expended significant quantities of sonobuoys, torpedoes, aircraft sorties, and ships hunting the other submarines. On several occasions, Argentine torpedo failures proved to be the only thing that prevented successful submarine attacks on Royal Navy ships. Although the Royal Navy never located the other two submarines, their efforts proved it was easier to kill submarines in port than at sea.
Defeating submarines in port is more aggressive and highly escalatory if done during peacetime. However, diplomacy and sanctions can restrict the flow of required parts and supplies, affecting readiness and preventing a submarine’s departure from port. Such strategies are hard to implement for adversaries with strong indigenous supply chains. During wartime, special operation raids and time-sensitive targeting can help disable vessels in port.
Infrastructure strikes on piers, warehouses, fuel storage, ammunition magazines, and communication nodes can hobble sustainment capabilities. An enemy submarine can return to port but cannot be redeployed. Mining the entrances or approaches to harbors can quarantine the threat, at least temporarily. Defeating submarines in port simplifies theater ASW and enables logistics to flow into the theater with less risk.
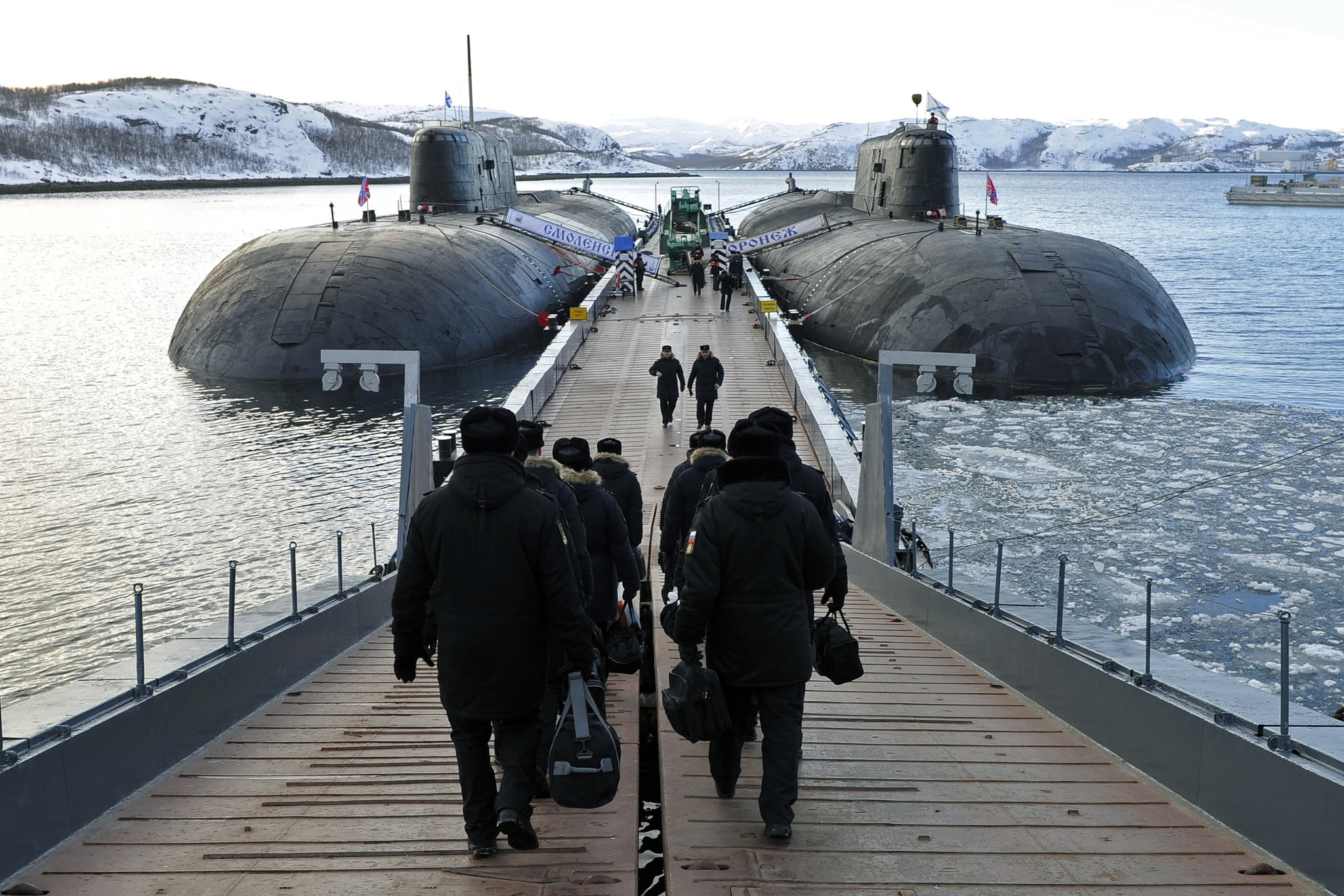
Operational Level of War
Anti-submarine warfare (ASW) is a resource-intensive fight. The time to learn and prepare is before hostilities break out. The combatant commander should support joint operations at the operational level of war by prioritizing and resourcing ASW as a key mission and emphasizing routine training requirements. ASW exercises illuminate the gaps in coverage within the operating area. Once those gaps are identified, the command can submit an urgent needs request to acquire additional capabilities to support the ASW mission. Urgent is relative to the budgeting time frame, so the joint force commander should identify these needs early to build a case for how these capabilities are required in the theater.
3. Defeat the submarines’ shore-based command-and-control (C2) capability. Severing leadership’s communications with their operational units ranges from destroying nodes, jamming channels, hacking command and control systems, and targeting leadership. Submariners embody mission command, but disrupting the command-and-control capability reduces the effectiveness of over-the-horizon targeting. If the enemy submarine does not have third-party sensor cues for the location of our ships, the submarine is forced to approach the strike group for organic acquisition. As a submarine draws closer to a protected entity, its advantages are eroded, and its chance of discovery is elevated. Disrupting communications also adds a layer of distraction, forcing adversarial crews to make efforts to restore communications.
4. Defeat submarines near ports and in denied areas. In the late 1940s and early 1950s, anti-submarine aircraft operated in spotter-killer groups. One carried the sensors, and the other carried the torpedo. Today, General Atomics builds the sensor-laden MQ-9B capable of carrying side-scanning maritime radars and 40 sonobuoys. Another unmanned aerial system, the T600, successfully launched a Stingray torpedo during a NATO exercise. While U.S. maritime patrol aircraft like the P-8A Poseidon are adept submarine hunters, there are too few of them.
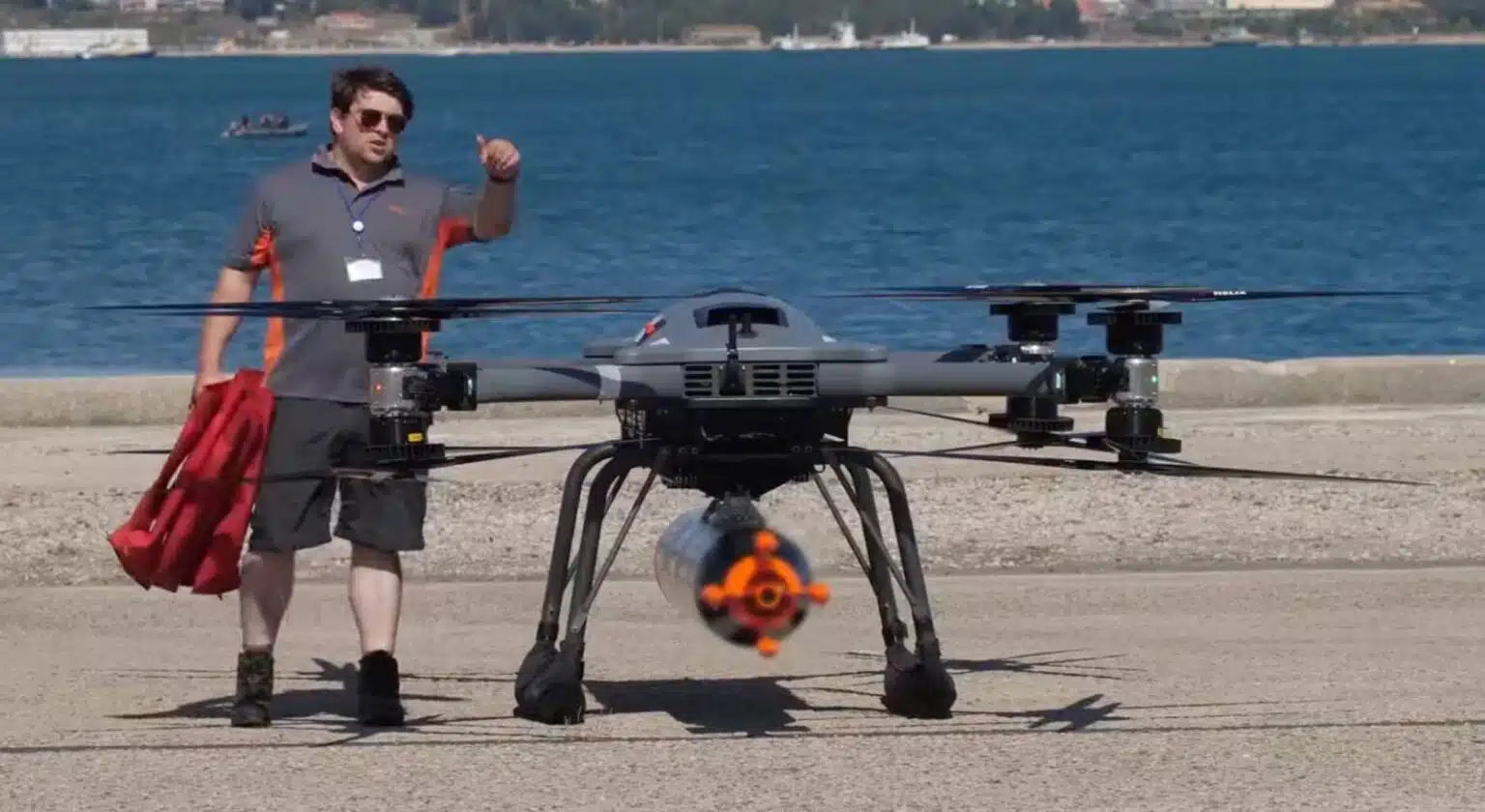
Hunting submarines is an excellent area where the joint force can shine. The Marine Corps should invest in the MQ-9B to support their Marine littoral regiments’ domain awareness and anti-submarine warfare initiatives. These aircraft could operate from expeditionary advanced bases near a hostile country to detect, track, classify, and engage hostile submarines.
5. Defeat submarines in chokepoints. Chokepoints are dangerous for warring sides depending on who has the preponderance of forces and who can position first. Diesel-electric submarines are slow and cannot easily close on a maneuvering carrier strike group. Instead, diesel-electric submarines prefer to lie in wait. Chokepoints are key maritime terrain to funnel forces and favor ambush predators. This is an area where the Marine Corps littoral regiments and the Army multi-domain task forces can play a decisive role with the proper weapons.
The Marine Corps littoral regiments and multi-domain task forces expect to operate near chokepoints, providing sensors and fires for the maritime fight. Equipping them with weapons and sensors that support anti-surface and submarine warfare would increase their lethality and utility in chokepoints. Equipping these units with MQ-9B Sea Guardians would provide persistent maritime domain awareness for the joint force. The Department of Defense should develop an extended-range dual-use anti-submarine/anti-ship cruise missile for the joint force like the Russian SS-N-14 Silex. These units could rely on inorganic sensors or be equipped with periscope detection radars, sonobuoys, and sonar processing systems to direct their maritime fires.
These units are already equipped with anti-ship missiles such as the SM-6, Block V Tomahawk, and Naval Strike Missile (NSM). Increasing the variety of weapons and capabilities makes these forces more lethal and forces the enemy to develop countermeasures, diverting resources from offensive weapons.
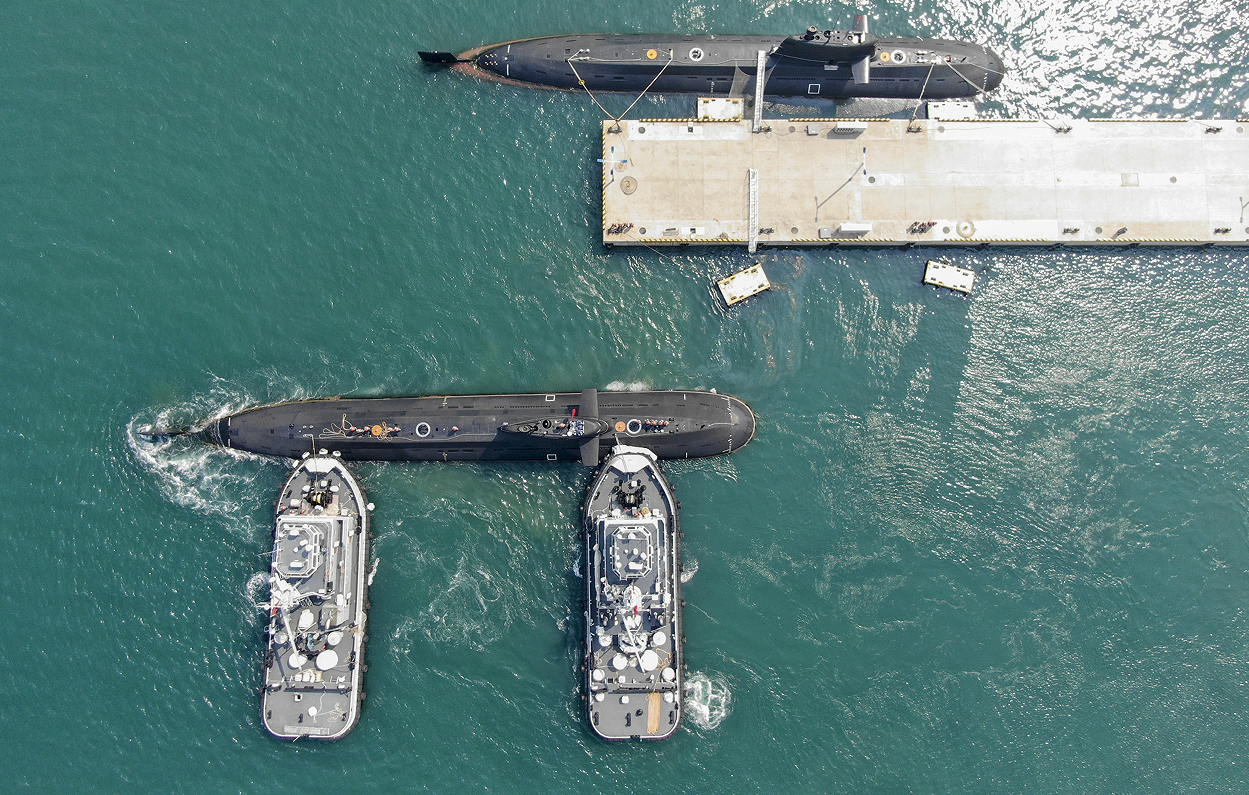
6. Defeat submarines in the open ocean. There are two key ways that the joint force can support the open ocean ASW fight. One is through providing additional intelligence support. Cryptologic Task Group (CTG) 101 is collocated with the Air Force, Space Force, and other agencies. It provides timely, accurate, and relevant target quality data on dynamic targets to enable weapons engagement at range. If the next fight is maritime, CTG 101 mission priorities should be increased to provide improved targeting and tracking of opposing submarines.
The other way to support ASW in the open ocean is by utilizing MQ-9Bs to support long-range maritime patrols, and there is precedence. During the Second World War, the Army Air Corps utilized B-17s and other aircraft to support ASW patrols from Iceland to the Caribbean to deter German submarines from surfacing. Air Force battle management and other special mission aircraft could play a similar role in finding submarines. Air Force “Compass Call” aircraft could support jamming enemy submarine communications, targeting, and scouting channels. Most submarine over-the-horizon targeting comes from satellite communications. Without those communications, the range of a submarine-launched missile is reduced to the organic targeting distance. This potentially reduces the range from 300 nautical miles to under 50 nautical miles. At those ranges, a carrier strike group’s organic ASW assets have a chance at locating and defeating an enemy submarine.
7. Draw enemy submarines into ASW “kill boxes.” Drawing the enemy into “kill boxes” utilizes joint force capabilities. The theater anti-submarine warfare commander should design kill boxes based on chokepoints, bathymetry, acoustic profiles, and the location of ASW-equipped friendly forces. Integration should be based on sensor and weapons capabilities.
8. Mask our forces from submarine detection or classification. There are several ways that the Navy masks forces from submarine detection or classification. Some of the easiest ways include operating in areas of the ocean with a large amount of acoustic noise, in areas with poor acoustic conditions for the spread of noise, and operating the engineering plant to present different acoustic profiles. Air Force aircraft could drop large noisemaking decoys offset from naval assets to drive enemy submarines away from the naval force. These self-propelled noisemakers are already in the Navy’s inventory as training tools. Noisemakers could also be configured to serve as lures, mimicking the acoustic profiles of worthwhile targets to entice submarines into kill boxes for joint fires prosecution.
Tactical ASW
The joint force plays a role in tactical anti-submarine warfare. The joint force assets used to detect, classify, and track submarines in chokepoints, the open ocean, and kill boxes must also be able to engage the submarine. Equipping littoral regiments and multi-domain task forces to engage submarines is vital as it might represent the last opportunity before the submarine can break into the open ocean.
Task forces comprised of escort carriers played several roles during the Battle of the Atlantic, escorting convoys or participating in hunter-killer groups. World War II task forces utilized signals intelligence to locate and attack German U-boats. Modern reincarnations of the hunter-killer group might include allied frigates, destroyers, and composite squadrons specialized for ASW. For example, the Japanese Mogami class frigate and squadrons composed of MQ-9Bs or MH-60R helicopters operating from Navy flattops like amphibious assault ships or expeditionary sea bases. Queuing would be supported by the federated intelligence apparatus in addition to the organic scouting capabilities of the group.
9. Defeat the submarines in close battle. With the advent of anti-ship cruise missile-armed submarines, the close battle can be anywhere from a few hundred yards to over 300 nautical miles. Supporting the force with ASW-capable aircraft, deploying missile countermeasures, jamming submarine communication and datalink capabilities, or providing intelligence of a missile launch must not be overlooked as meaningful contributions by the joint force. This weaves into the next thread.
10. Defeat the incoming torpedo. This thread should be updated to include defeating incoming missiles and torpedoes. If all else fails, defend. This thread is primarily a local force action, but there are ways the joint force can still contribute. Mines and submarine nets deployed by littoral regiments and other forces around chokepoints or vulnerable waterways can complicate adversarial targeting. Utilizing soft kill options like decoys or tactical air defenses to engage a missile at range can help thwart a long-range missile attack. Lastly, the joint force can provide indications and warnings of adversarial weapon launches to help friendly assets prepare to defend.
Conclusion
The ten threads of full-spectrum ASW provide an excellent path for joint force integration into the ASW Fight. Some retooling may be required to become effective. As the joint force girds itself for a maritime fight, new units in development to support local sea control or denial should not overlook ASW and invest accordingly.
As in the Second World War, defeating the submarine threat would require a whole-of-government approach in close coordination with allies and partners. It is better to start communicating and training for anti-submarine warfare during peacetime rather than unnecessarily expending blood and treasure in wartime. As the military gears up to fight a maritime fight in the Pacific, every service is eager to play a role. There are roles for the joint force, and the U.S. Navy should take advantage by steering other service resources in ways that improve the Navy’s lethality and help win the ASW fight.
LCDR Jason Lancaster is a Surface Warfare Officer. He currently works at OPNAV N5. His last sea tour was as C5I Officer aboard USS AMERICA (LHA 6). Afloat he has also served as a Destroyer Squadron Operations Officer, Weapons Officer on a DDG, with division officer tours aboard an LSD and an LPD. Ashore, he has worked in the N5 at OPNAV and Commander, Naval Forces Korea. He is an alumnus of Mary Washington College and holds an MA in History from the University of Tulsa.
The views presented are his own and do not necessarily reflect the official view of the U.S. Government or the Department of Defense.
Featured Image: A PLA Navy submarine attached to a submarine flotilla under the PLA Northern Theater Command bears off a port for the maritime combat training drills on March 23, 2022. (Photo via eng.chinamil.com.cn/photo by Wu Haodong)