Redefining Readiness Topic Week
By Connor S. McLemore, Shaun Doheney, Philip Fahringer, and Dr. Sam Savage
On April 24th, 1980, eight American helicopters heavily laden with special forces and their equipment launched from the aircraft carrier USS Nimitz operating in the Arabian Sea. They flew northeast into the Iranian desert to rendezvous with refueling aircraft in order to attempt a rescue of 52 hostages taken in 1979 from the American Embassy in Teheran. The operation, Eagle Claw, ended in disaster: a dust cloud kicked up by aircraft propellers and helicopter rotor blades caused one of the helicopters to collide with a refueling aircraft and explode, killing eight U.S. personnel and wounding several others. Yet the mission had already been aborted prior to the collision. During the flight to the refueling site, three helicopters suffered equipment failures, leaving just five able to continue the mission. Mission go/no-go criteria required at least six helicopters to continue, and the order from the president to abort the mission was passed. The tragic collision occurred when aircraft were attempting to transfer fuel in order to depart Iran after the mission was already cancelled.
Helicopter capability to support Eagle Claw was quantifiable based on historical helicopter failure data, and yet prior to the mission, it was not quantified. The Holloway Report, which detailed the results of the investigation into the mission’s failure, laid bare how the number of helicopters sent was a major contributing factor to the early mission abort, and recommended that more helicopters should have been sent. Using basic probability theory and known helicopter failure rates, the mission had an estimated probability of 32 percent that there would not be six helicopters ready at the refueling site. Former President Jimmy Carter said in 2015, when asked if he wished he had done anything differently as president, “I wish I’d sent one more helicopter to get the hostages, and we would have rescued them, and I would have been re-elected.” Yet over 40 years later, the same underlying military readiness shortfalls that prioritized availability over capability for those helicopters remain largely unfixed.
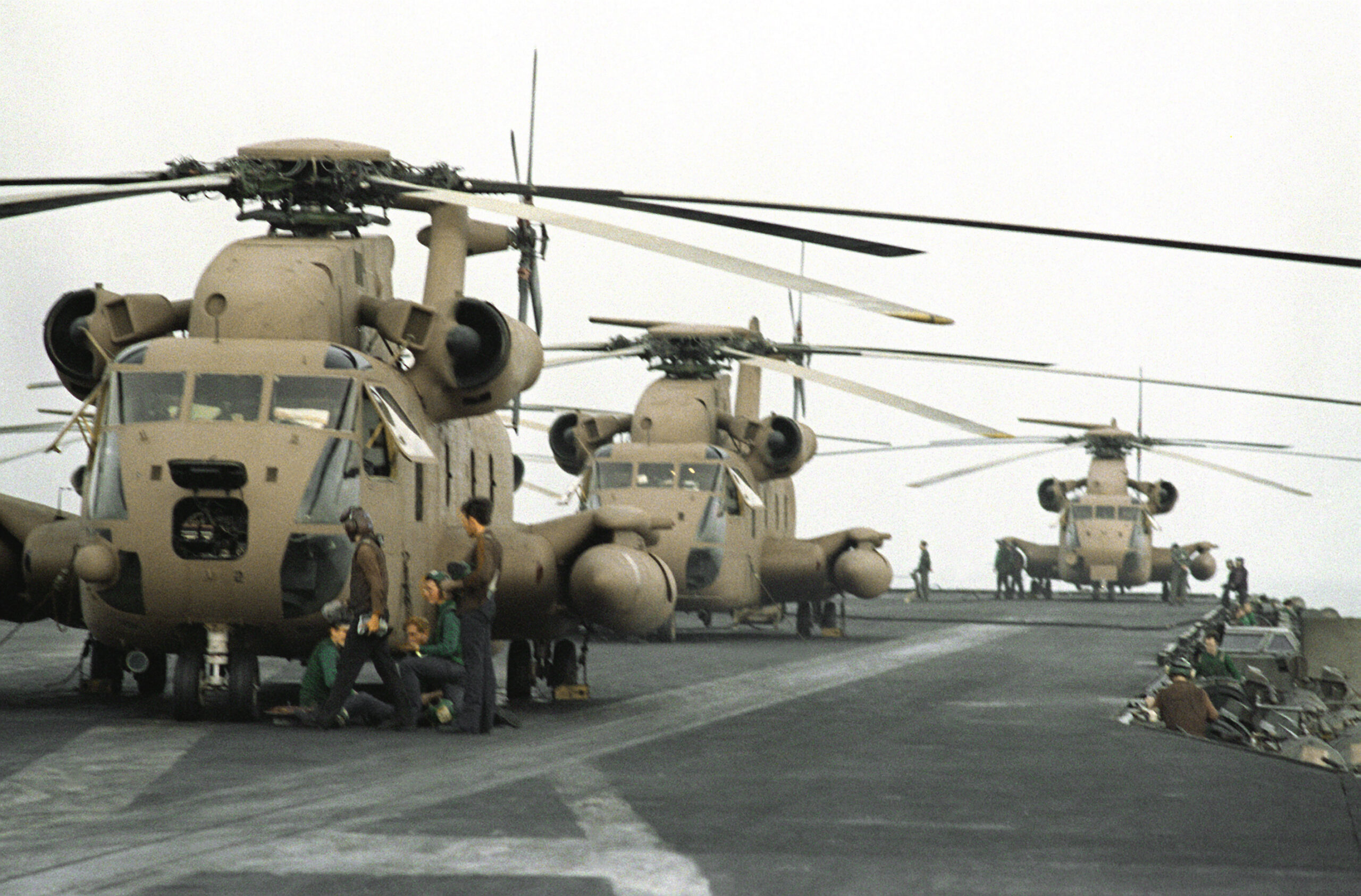
The urgent need for changes to the military’s existing readiness framework has been called for by General Charles Brown, the chief of staff of the Air Force, and General David Berger, the commandant of the U.S. Marine Corps. In their recent War on the Rocks article, they describe the necessity of a better analytical framework for the joint force to better assess balancing operational costs of existing forces with investment costs to modernize and replace those forces. The two service chiefs point out, “Our current readiness model strongly biases spending on legacy capabilities for yesterday’s missions, at the expense of building readiness in the arena of great-power competition and investing in modern capabilities for the missions of both today and tomorrow.” In order to address that problem, they call for “a framework for readiness” and “a more precise understanding of risk — to what, for how long, and probability.” Our team at Probability Management, a 501(c)(3) nonprofit dedicated to improving communication of uncertainty and risk, wholeheartedly agrees.
Achieving the service chiefs’ vision of a better analytical framework will require changes to both the qualitative and quantitative underpinnings of the existing readiness system. To improve the quantitative parts, we recommend the implementation of a supporting data framework that is capable of informing probability-based capabilities assessments by making the Defense Department’s readiness data more flexible, visible, sharable, and usable.
Diagnosing the Problem
The readiness system contributes to unquantified capabilities of combinations of military assets, zero-risk mindsets in combatant commanders, and requirements that are excessive. These problems must be addressed in any future readiness system. It is unreasonable to expect service chiefs to push back on requirements from combatant commanders if discussions around the capabilities of combinations of military assets are purely subjective. We make no claim as to what acceptable capability thresholds should be; however, we must point out that even if the service chiefs and combatant commanders were in complete agreement on a threshold, without a way to quantify the probability of achieving it, requirements will probably remain excessive. Requirements supported with too many or not enough forces leads to imbalanced risks and costs. Why pay to achieve a 99.9 percent chance of success when a 90 percent chance of success is adequate? Conversely, why risk a 10 percent chance of failure when a 99.9 percent chance of success is required? The existing readiness system does not support thinking in these terms.
Fixing the limitations of the existing readiness system is not purely a data challenge. However, too many problems with the existing military readiness system are a direct result of the ways in which Defense Department data is being collected, stored, and communicated. We do not advocate for the military readiness system to remove subjectivity from all readiness calculations – the readiness system should always endeavor to support the service chiefs in assessing what is meaningful, not just against what is measurable. However, subjectivity supported by systematic estimates of probability is likely to outrun subjectivity alone. The existing readiness system is simply not capable of providing military leaders with timely, fully-informed systemic probabilistic estimates of mission capabilities.
A principal problem with the existing system is that metrics associated with readiness requirements are routinely measured as “ready or not.” Once a unit meets a defined level of performance, the unit is declared “ready.” However, even when units are “ready,” it is often vague as to what they are ready for and when. Additionally, the percentages used in current readiness metrics cannot easily be aggregated by mathematically defensible means. For example, a notional requirement could be that at least 80 percent of unit systems must be ready. If a unit with 90 percent of its systems ready reports as “ready,” and pairs with a dissimilar unit at 70 percent and reporting “unready”, the combined capability of the two units to support a given mission at an uncertain future time is unclear. Fundamentally, the existing military readiness system cannot be used to quantitatively predict probabilities of mission success at uncertain future times for portfolios of dissimilar assets.
When joining readiness levels of dissimilar units, the lack of a mathematically defensible readiness framework results in important issues being distorted or lost, leaving little coherence for understanding the capabilities of the joint force at the operational or even strategic levels. Because joint force capabilities required by combatant commanders are not being credibly quantified, and because the service chiefs, who are tasked with providing ready and capable military forces to combatant commanders pay to support those requirements, the combatant commanders have little incentive to ask for fewer forces than are needed. Additionally, combatant commanders are responsible for requesting capabilities that cover their missions now, not several years in the future. Why would they risk failure by not requesting enough forces, especially considering they aren’t paying for the forces they receive? Combatant commanders are simply doing their jobs when they prioritize getting more forces in their theater now over future capabilities. Yet this is clearly a problem because marginal improvements to fulfil near-term requirements may be coming at enormous cost to important future capabilities.
DoD is not being hindered by a lack of enough data. Rather, it is hindered by an inability to see and make use of the data it collects in its many vast, opaque, stovepiped databases. Today, most military readiness data arrives in the form of historical records and subject matter expert opinions. An ideal future military readiness data framework should be able to better use that existing data while also enabling the continuous and increasingly automated collection and sharing of additional data sources that are authoritative, timely, clean, and contain useful information. Yet it is simplistic to think it will be possible to efficiently apply advanced data science techniques such as simulations, regression, machine learning, and artificial intelligence on data that is not easily visible, sharable, and usable. The lack of the appropriate data framework prevents a readiness framework from being informed by timely, visible, usable data. Fundamentally, there is no format today that allows for the efficient sharing of datasets in DoD, and much time is being wasted figuring out what data is available, if it contains useful information, and then transforming it manually so that it can be used for analysis.
A New Framework
A real gap lies in the ability of DoD to quantitatively measure what makes military units combat effective (vice combat available) and the associated costs of those capabilities. We propose the military adopts a uniform data framework that could be used within and across military services and systems to better quantify readiness predictions both now and in the future, such as providing timely estimates of the probability that helicopters will not be capable for a given mission, and to better communicate risks, such as the probability of an important mission failing because not enough helicopters are being sent. Such a data framework would allow for better sharing and employment of existing data, resulting in better quantitative metrics, leading to better cost and risk tradeoff discussions among decision-makers. This could be used to allow decision-makers to see forward in time as well with capability outcomes generated continuously through the use of large military datasets. This will allow data sources and models to evolve over time, resulting in improving probability-based capability predictions that would go a long way toward supporting the outcomes Generals Brown and Berger propose. A new approach can allow planners, commanders, and decision-makers to speak the same language to communicate, “How ready are units for what?”
Probability Management has long advocated for improvements to the quantitative underpinnings of the military readiness framework, and detailed technical explanations and example use cases for the data framework we recommend can be found in our published technical articles. In our work, we describe the underlying problems of the existing framework, and describe necessary steps if the joint force is to adopt a “holistic, rigorous, analytical framework to assess readiness properly,” as the service chiefs rightly demand.
In straightforward terms, the data framework is best explained as the standardized representation of the readiness of military assets in the form of columns of data with statistical dependence between columns preserved. Unlike data in the existing framework, these columns of data can then be straightforwardly rolled up to probabilistically estimate the capabilities of groups of dissimilar assets operating in uncertain environments. This data framework can be used to improve military readiness reporting systems broadly by conveying the probability of achieving specified levels of availability and capability for specified missions now and at uncertain future times. We believe the limits of the existing system do not result from the limitations of math, but rather from the limits of the data structures employed in readiness calculations. In contrast, our framework supports simple and straightforward arithmetic, while transparently carrying along probabilistic information that may be extracted when required.
The basic approach, democratized and standardized by Probability Management, does not forecast the future with a single number, for example, “on average a helicopter is operable 75 percent of the time,” but instead models many possible futures for each helicopter using cross-platform data standards. In terms of Operation Eagle Claw, consider eight columns of data with 1,000 rows each, with each column of data representing one helicopter. Every row represents a different possible future, with a one in a row if the aircraft is up and a zero if it is down. Each row will have on average 750 ones and 250 zeros. The total number of operable aircraft out of the eight is represented in a ninth column summing each of the original eight columns row by row. When the original Eagle Claw assumptions are entered into this framework, of the thousand elements in the ninth column, about 320 (32 percent) would have fewer than the six required for the mission, indicating a 32 percent chance of failure. These sorts of row-by-row calculations, known as vector operations, are trivial in virtually any software platform today, so the open standard framework could be used to enable chance-informed decisions across any other existing or future readiness software platforms.
When the readiness of an asset is represented as a column of thousands of “ready or nots,” then units can be combined in a row-by-row sum to provide a column of assets available in each of 1,000 futures. This approach, long used in stove-piped simulations, becomes a framework by simply storing simulated results in a database and making them sharable.
For example, suppose the DoD is choosing between two aircraft systems, A and B. They are both operational 75 percent of the time, but A is 5 percent cheaper than B. We might pick A to save money. But suppose that system A goes down at random 25 percent of the time, and system B is guaranteed to be operational for 7.5 flight hours and then require 2.5 hours of maintenance. Traditional readiness metrics based on averages can’t detect the difference between A and B, except on cost. But for missions requiring less than 7.5 flight hours, system B is vastly superior, because you can arrange to have 100 percent of the fleet in action. The added predictability may be well worth the 5 percent cost premium. For missions over 7.5 hours, system B is worthless, as no aircraft will be ready. With System A, however, a few planes will survive the long mission. So again, we should be asking “how ready for what,” where “how ready” may be interpreted as “what are the chances?” Chance-informed capability decisions would allow the Defense Department to quantify cost today versus the chance of adverse events tomorrow.
Conclusion
The adoption of this new data framework for military readiness would go a long way toward achieving the quantitative underpinnings necessary to support the service chiefs’ vision and it can be used to fix the fundamental problem they call out: the “gold-plating” of existing force requirements at the expense of future capability. Additionally, the framework we propose is merely a data standard, not requiring any particular software implementation, is not proprietary, is available at no cost to the government, and does not require the wholesale elimination of the existing military readiness system; it can expand upon the existing system and be implemented incrementally. It is not designed to eliminate subjectivity from a commander’s readiness calculations, nor should it. A more structured readiness approach that explicitly acknowledges uncertainty is complementary to subjective estimates. Commanders will still need to make decisions subjectively based on myriad factors, including their own risk tolerance.
Combining the predicted risks of a portfolio of dissimilar assets occurs commonly in the commercial sector. Our approach has long been widely used in applications in financial engineering, insurance, and many other industries. It has been applied to portfolios of oil exploration projects at a global energy firm and portfolios of risk mitigations at a large utility. We are confident that our approach is both straightforward to understand and simple to use without specialized software or mathematical training. For example, using the same data framework we propose the military adopts for its readiness system, Probability Management taught modern portfolio theory, a complicated subject that involves evaluating the predicted returns of financial portfolios, to West Oakland Middle School eighth graders in 2017. The students quickly understood the framework and employed it effectively, and we are confident that military personnel will be able to easily employ it in the area of military readiness.
Our proposed data framework is already adopted by commercial organizations in sectors as diverse as healthcare, energy, and defense to quantitatively support decisions and mitigate risks. Lockheed Martin, Pacific Gas & Electric, and Kaiser Permanente are incorporating the framework to better assess the likelihood of critical outcomes in terms of probabilities of success and failure based on historical performance and future predictions.
The data framework we propose is generic and is easily tailored to new-use cases and industries, including to an improved military readiness framework. The expectation is that if our approach were applied in a military readiness context, it would support a better analytical framework for the joint force and allow better assessments. This would help better balance operational costs of existing forces with investment costs to modernize and replace those forces. Through this framework, military leaders would have a more practical understanding of the tradeoffs within military readiness and better manage the challenges of today and tomorrow.
Mr. Connor McLemore is a principal operations research analyst for CANA Advisors and the Chair of National Security Applications at ProbabilityManagement.org. He has over 12 years of experience in scoping, performing, and implementing analytic solutions. He holds Masters’ degrees from the Naval Postgraduate School in Monterey, California, and the Naval War College in Newport, Rhode Island, and is a former naval officer and graduate of the United States Navy Fighter Weapons School (TOPGUN) with numerous operational deployments during 20 years of service.
Shaun Doheney is a Senior Data and Analytics Strategy Consultant for a large global company with experience as a Chief Analytics Officer for an Inc. 5000 company. He is a retired Marine Corps Lieutenant Colonel who has conducted, participated in, or led a whole host of analyses and evaluations across major Department of Defense decision support processes. He is the Chair for the Military Operations Research Society’s Readiness Working Group and the Chair of Resources and Readiness Applications at ProbabilityManagement.org.
Mr. Philip Fahringer is a Fellow and Strategic Modeling Engineer for Lockheed Martin Aeronautics with 35 years of combined military and defense applied research in analytics and decision support. He holds a Master’s degree Operations Analysis from the Naval Postgraduate School in Monterey, California, and in Strategic Studies from the Army War College in Carlisle, Pennsylvania, and he is a former naval officer with numerous operational deployments and strategic planning assignments during 20 years of service.
Dr. Sam L. Savage is Executive Director of ProbabilityManagement.org, a 501(c)(3) nonprofit devoted to the communication and calculation of uncertainty. The organization has received funding from Chevron, Lockheed Martin, General Electric, PG&E, Wells Fargo and others, and Harry Markowitz, Nobel Laureate in Economics was a founding board member. Dr. Savage is author of The Flaw of Averages: Why We Underestimate Risk in the Face of Uncertainty (John Wiley & Sons, 2009, 2012), is an Adjunct Professor in Civil and Environmental Engineering at Stanford University and a Fellow of Cambridge University’s Judge Business School. He is the inventor of the Stochastic Information Packet (SIP), a standardized, auditable data array for conveying uncertainty. Dr. Savage received his Ph.D. in computational complexity from Yale University.
Featured Image: NORTH PACIFIC OCEAN (May 3, 2021) – U.S. Marine Corps MV-22 Ospreys, assigned to Marine Medium Tiltrotor Squadron 164 (Reinforced), 15th Marine Expeditionary Unit, prepare to take off from the amphibious assault ship USS Makin Island (LHD 8) in support of Northern Edge 2021. (U.S. Navy photo by Mass Communication Specialist 2nd Class Jeremy Laramore)
The proposed system seems to have advantages, but there is always the human factor. Back in 1980 I was on Eisenhower heading to the IO to relieve Nimitz. There was huge pressure for the air wing to have at least an 80 percent full systems capability. But after flying aboard off Norfolk, the FSC level was around 65 percent. We were doing a 22 kt SOA through the South Atlantic, so no parts were going to be CODed aboard. LANTFLT kept pinging the battle group staff, and eventually they caved and started sending Norfolk numbers they wanted to hear rather than what was actually the case. Fortunately we never had to test the air wing via actual combat. Any statistical system is sensitive to human inputs, which are a function of sociology, not physics.
It is not exactly clear to me what framework it is that you are proposing. Your example of the number of working helicopters is relevant to the materiel readiness portion of a unit’s overall readiness, but the framework seems to be lacking a means to address other elements of readiness such as personnel readiness, levels of supply of materiel, or sufficiency of training. Perhaps instead of measuring how many people or major end items a unit has, we should instead start to measure how well the unit performs its assigned tasks, such as how quickly it can close a kill chain (combat arms) or how many supplies it can deliver in a set time period (logistics)? Changing what is measured changes the focus. We still need to make sure units have sufficient numbers of people and equipment, but putting the emphasis on and measuring performance of assigned tasks would provide a better representation of how a unit would perform when employed and encourage service members and commanders to innovate and try new ways to improve performance with whatever tools they have available.
Hi Julia, thanks for the comments. A major benefit of the data framework we propose is that it does allow the sorts of dissimilar readiness types you mention to be combined straightforwardly. We are agnostic about what the military actually chooses to measure but we do think a data framework used should be flexible enough to support whatever is measured. I would point you towards one of our more technical articles that describes in detail how you would use the data framework to meaningfully combine different readiness types. https://static1.squarespace.com/static/5a4f82d7a8b2b04080732f87/t/5dfc054b93c3160dfed60c91/1576797517608/Phalanx-Volume-52-No-4-Calculating-Carrier-Air-Wing-Readiness.pdf